5 steps to building data literacy within L&D
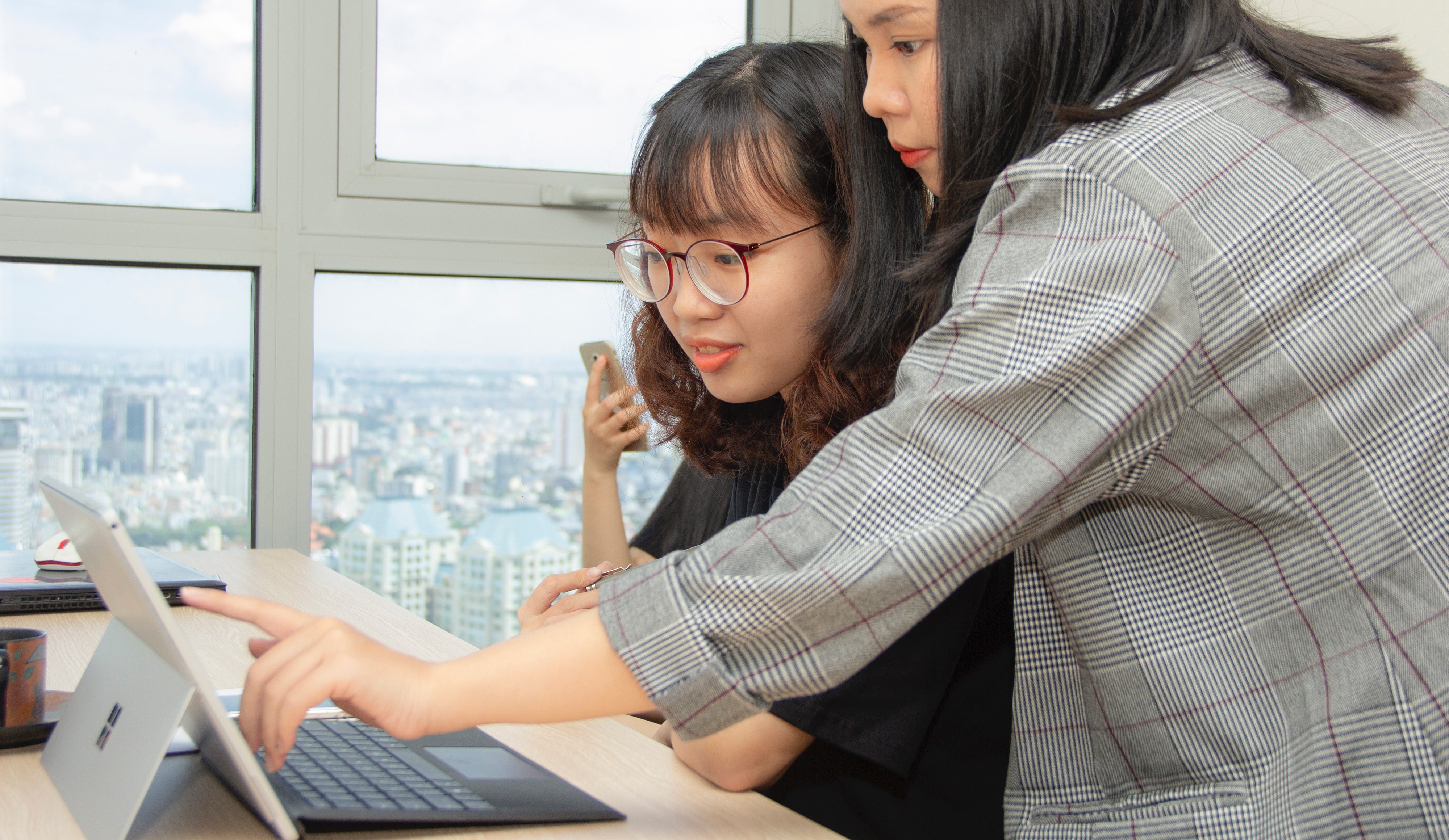
We’re living in a data driven world. Due to machine learning, robotics and the automation of almost every simple task, data is driving our lives in ways we cannot fully comprehend or control. However, with regards to learning programs, employee performance, and achieving business goals, there are finite and meaningful data sets that L&D professionals should examine for insights and use to inform decisions.
Data literacy is a term being used more often lately to describe a common language used by business people to communicate about data.
Gartner defines data literacy as “the ability to read, write and communicate data in context, including an understanding of data sources and constructs, analytical methods and techniques applied — and the ability to describe the use case, application and resulting value.”
Data literacy allows operations leaders to communicate success measures to the c-suite. It helps finance convey urgency when the sales function is not meeting quarterly and year targets. It allows L&D to communicate quickly and effectively what it does and what impact it has for the business. The Learning Report 2020 indicates that L&D is not effectively communicating impact to business leaders. Data literacy facilitates the story telling processes by mastering some measurement basics.
Here are five things L&D should do to build data literacy:
- Know your data.
There are so many types of data that it is essential to know what you are working with. Surveys often produce Likert scale data based on a 5-point scale and open-ended questions which produce qualitative data (comments). Learning Management systems produce transactional data—cases and counts of the number of people trained, which courses were completed, and how many people are compliant. Another type of data, business outcome data, might include sales forecasts, actual sales figures, or margin values.
Build literacy by understanding the parameters of the data. Likert scale data will be limited to the values on the scale. On a 5-point scale, there should only be whole number values from 1 to 5. Values of 0, 6 or anything else are anomalies. LMS and business/sales data are likely to be more complicated, so it is important to understand what is normal for the data you are investigating. To understand “normal” it helps to understand some basic statistics, which we’ll cover in the next section. - Describe your data.
Generally speaking, there are two types of statistics: descriptive and inferential. We’ll focus on descriptive stats here and leave the inferential (also known as predictive) stats to the professional number crunchers. As the name implies, descriptive statistics use numbers to describe a data set. You probably know most of them but may need some brushing up on what they describe. Mean (average), median (middle most score in a rank ordered list) and mode (most frequent score) are measures of central tendency—middle-ness. Why middle-ness? It's a basic assumption that the middle-most score best represents all scores. Spread is the next important stat and it describes how wide the data stretches around the middle-most point. Variance and standard deviation are commonly used terms. These formulas are a bit complex but two important characteristics are desirable: 1. The data should be distributed normally—that is, in a bell shaped curve around the average score and 2. A smaller spread is usually better than a wider spread. It means that the average score is more precise and represents all scores well. If a 50 is the average of three scores 49, 50 and 51, the spread is small and 50 represents 49 and 51 equally well. If 50 is the average of three scores—0, 50 and 100, then it does not represent 0 or 100 well.
It is also important to know other descriptors like the number of people responding to survey (N) or the number of cases included in the analysis. Using the mean, standard deviation, minimum, maximum and N, a person can easily describe a data set in a way that others can understand. - Compare your data.
Simple statistics can be helpful to describe a data set, but it is often more insightful to compare two data sets. Consider two groups of electrical engineers who attend a course on applying principles of magnetism to generate electricity. A group of experienced employees might rate the course low for the question about learning new knowledge and skills whereas new employees might rate it high. The comparison shows a difference. - Draw conclusions.
Statistical analysis alone is not enough. To provide insights you must examine the data and figure out what is happening. With the engineering example, experienced engineers had already gained the information taught during the course while working on the job. The new group of engineers has not learned the information and therefore find the course valuable. The conclusions to draw are fairly simple. Experienced engineers already know the course information, therefore, they should not have to attend. Only new engineers should be required to attend. - Tell your story and take action.
When meaningful insights are uncovered, they only become valuable when someone takes action. With the engineering example, an L&D professional can take action. Advertise the value of the course for new engineers. Communicate to experienced engineers and their managers that the course is not required because the material is most likely already known. Create an advanced course for experienced engineers that focuses on different information that is not known but still applicable to the job. Exclude new engineers from this course until they have completed the first course. The actions are not complex. They do not require much thought, but they do require, well...action. The simple changes mentioned here will reduce scrap learning among experienced engineers and increase quality scores for both new and experienced engineers because both groups are getting the information they need.
How to get started
Creating a data literacy program should be a simple project not a monumental endeavor. While some of the content can be complex, like formulas and inferential statistics, the core content is easily understood by most learners. Here are a few suggestions for getting started:
- Identify the learning group and understand their needs. L&D is often a mix of business professionals and learning professionals. Most undergraduate and graduate programs require some credits in statistics, so the general groundwork has been laid. Assess the proportion of your target group that has knowledge and skills with data. If the percentage is low, start with the basics. If the percentage is high, skip the basics and move toward advanced analysis and how to take action with the results.
- Determine the right learning methodology(ies). Data literacy can be taught quickly as an in-person classroom course, but it also fits well as an online course with bite-sized topics, or as a virtual instructor-led course. While understanding the needs of the learners, also determine what learning methodology is best. Provide a mix of learning options if possible including courses, one-on-one coaching, data parties, and job aids.
- Build and test the content. After you build a portion of the content, begin sharing it with a group of potential learners. Get their feedback on the outline, methodology, content and exercises. Adjust based on feedback.
What content should you cover?
Here’s a quick outline that should be valuable:
- Common L&D measures, metrics, and data sources. Explain the different types of data collected and analyzed for L&D such as completion data from the LMS, cost data per program, Likert scale and comments data from learner surveys, and outcomes data from operations and finance systems.
- Key Performance Indicators (KPIs). Stay focused. Having too many metrics can be overwhelming for L&D professionals and business leaders. Focus on a small number of KPIs that are useful for stakeholders (e.g., instructors, programs managers, directors, business leaders). Only report the information that is relevant to each group.
- Common Reports. Most L&D professionals will not have to gather and report their own data. A system or an analytics team will provide results. L&D professionals need to gain familiarity with the standard reports they will receive.
- Interpretation and action. Once L&D professionals understand data basics and reporting basics, they will be ready for interpretation and action. Share guidelines for success that will help L&D professionals make decisions. For example, if the reports provide benchmarks, then suggest taking action only when scores fall below benchmarks. If benchmarks do not exist, provide guidance on scores that are acceptable. Also provide examples of reasonable actions they can and should take based on the results such as coaching instructors, revising exercises, scraping content, and including/excluding learner groups.